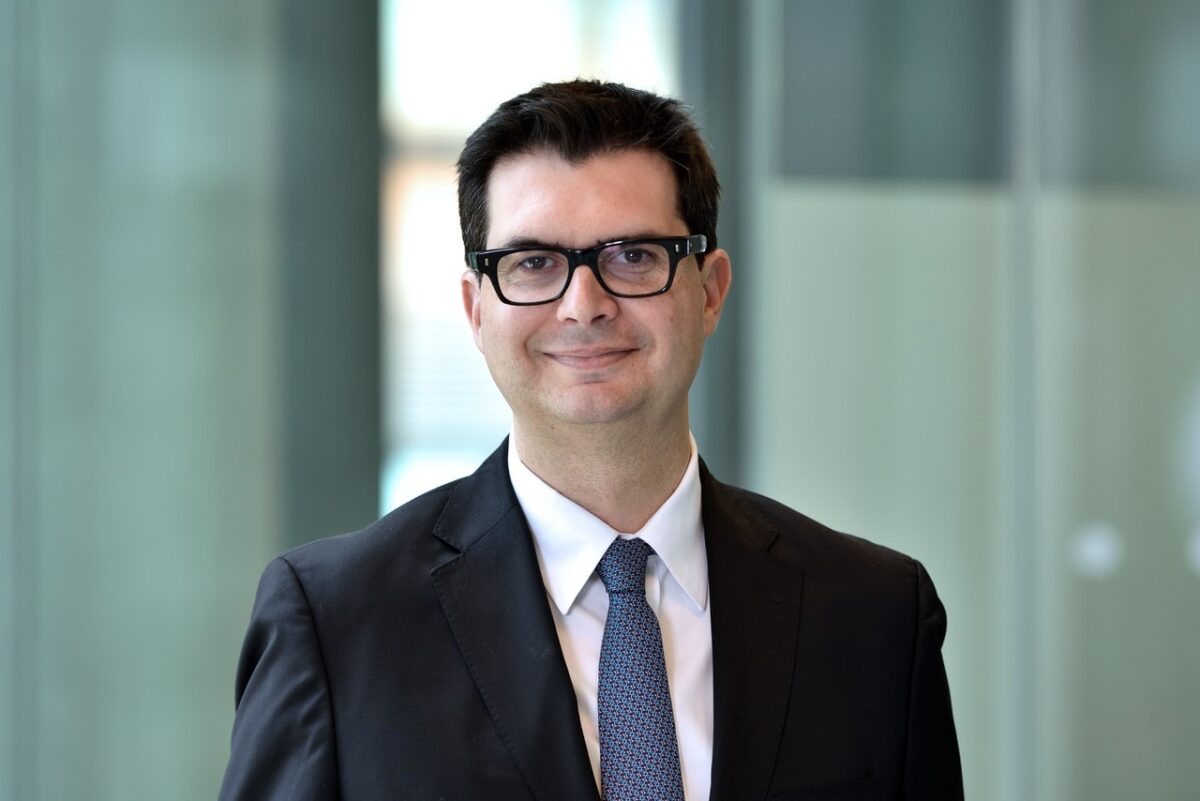
Shaolei Ren: “Global water cycle is out of balance”
Professor from the University of California, Riverside, investigates the water footprint and environmental impact of large AI models
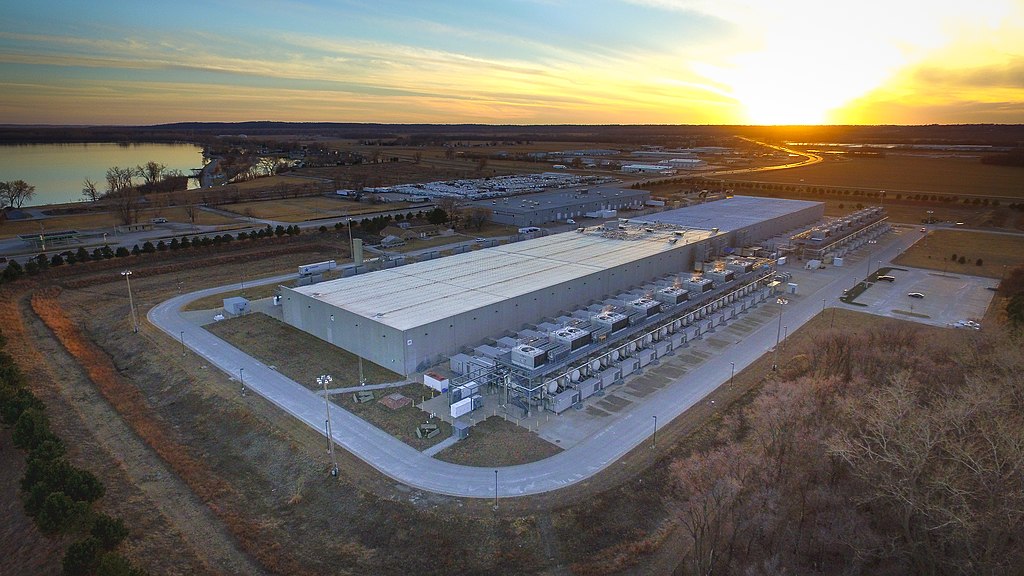
Artificial intelligence (AI) models are increasingly offering solutions to global challenges, including climate change and energy consumption management. Based on the premise that water is a finite resource, efforts are being made to transparently measure and understand the water and carbon footprints of AI.
At the same time, there is a drive to reveal the environmental impact of the AI models themselves in terms of the consumption of natural resources.
Research led by Shaolei Ren, a professor of electrical and computer engineering at the University of California, Riverside, is exploring the so-called “secret water footprint” of these systems.
According to Ren, the training of large AI models can consume millions of liters of water. However, the same technological advances could be used to reduce this consumption.
Studies carried out by Ren with the aim of revealing the secret water footprint of AI models indicate that it can take millions of liters of water to train a large AI model.
His work also indicates when and where AI itself could be used to reduce consumption. “For truly sustainable AI, we need to take a holistic view of water and carbon footprints,” Ren explains in an exclusive interview with Science Arena.
Science Arena – Why does the water footprint of AI still receive less attention than its carbon footprint? What can be done to change this?
Shaolei Ren – In addition to producing carbon, burning fossil fuels also generates a lot of atmospheric pollution, which directly impacts public health. Water is no exception. We need to educate society about what really happens when we consume water. When you take a shower, it uses a lot of water, but most of this water will be reused for other things. So you’re not really consuming that much water. Homes only consume or evaporate about 10% of water.
Another major factor is the use of water for agriculture and livestock farming. It takes a lot of water to produce a kilogram of meat. But 95% to 97% of it is “green” water contained in the earth, used by the plants that are fed to the livestock.
When we talk about AI’s footprint, we are also talking about heat and electricity. Water used for AI computing is mostly “blue water.”
It is fresh water from rivers and lakes, which humans could use directly. Industries are growing, the global water cycle is out of balance, and we need to conserve more.
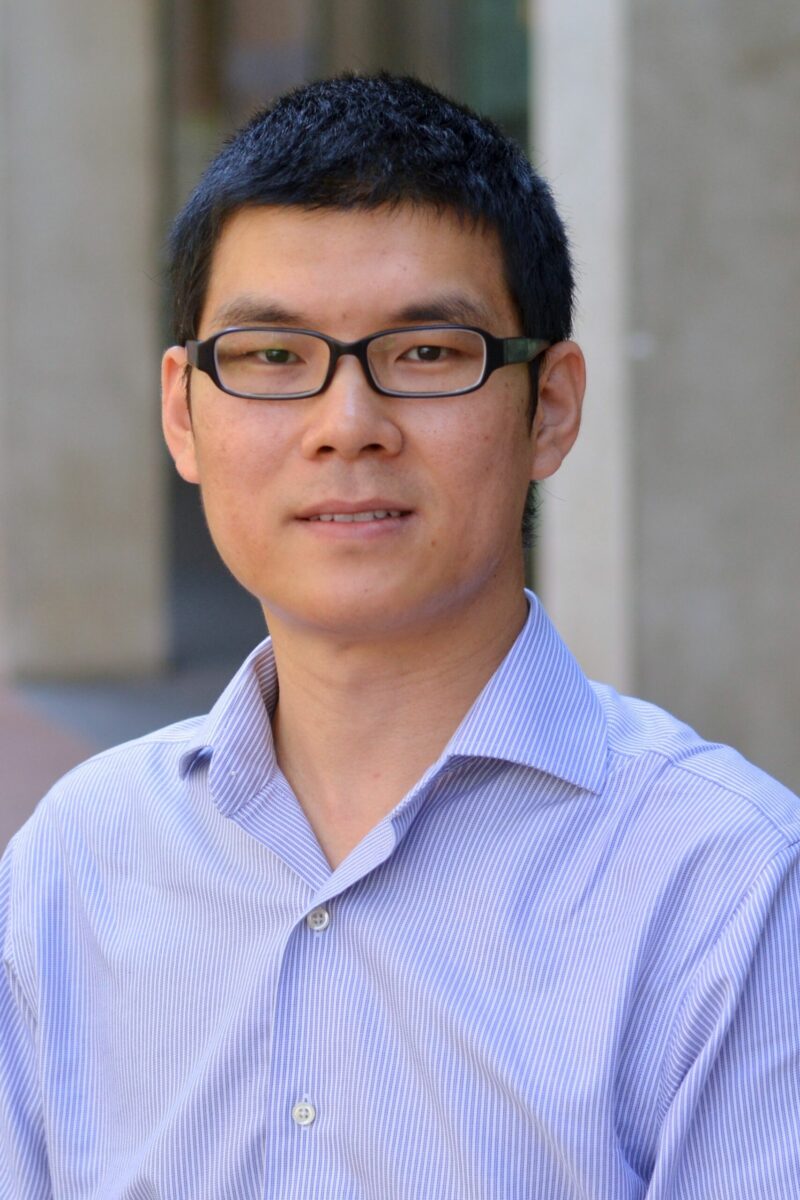
The training of GPT-3 was highlighted in your studies. Can you explain the water impact of large AI models with large data centers?
In the methodology we adopted, we estimated the total water footprint—considering withdrawal and consumption—including operational water and embodied water. We analyzed GPT-3, a model with 175 billion parameters used for language services.
The training of GPT-3 at Microsoft’s state-of-the-art data centers in the USA can consume a total of 5.4 million liters of water.
The concern is that these numbers will be even higher for GPT-4, launched in 2023. The GPT-3 model was trained a few years ago and is no longer considered particularly large.
Even in the larger language models, an individual inference does not require much energy or water. But considering the total demand, the total water consumption for an inference is higher than in the training phase.
Can GPT-4 itself predict future impacts on resource consumption?
There isn’t enough concrete data on GPT-4 yet, but we can make projections based on smaller models.
For example, the hydroelectric power needed for a system like GPT-3 to write an average email is equivalent to drinking a 500-milliliter bottle of water.
What measures could be taken to reduce the water footprint of AI models? Is it possible to balance the water and carbon impacts?
First, we need knowledge. We need to know what water consumption is so that we can make an informed decision. Then we need to look at different regions, considering water scarcity and efficiency. Tech companies are already doing carbon-intelligent computing.
Essentially, they try to shift their computing power to more carbon-efficient locations to reduce emissions. And they can do the same thing with water. Water is a regional resource.
Is there an effective way to balance water and carbon impact at the same time?
Understanding the impact of both carbon emissions and water consumption needs to be a common goal. To solve the math problem, both elements need to be in the equation. Local communities need to be represented in the political arena so that water doesn’t get neglected. In places like Uruguay and Arizona, where they are facing severe droughts, it is essential that water is prioritized.
*
This article may be republished online under the CC-BY-NC-ND Creative Commons license.
The text must not be edited and the author(s) and source (Science Arena) must be credited.