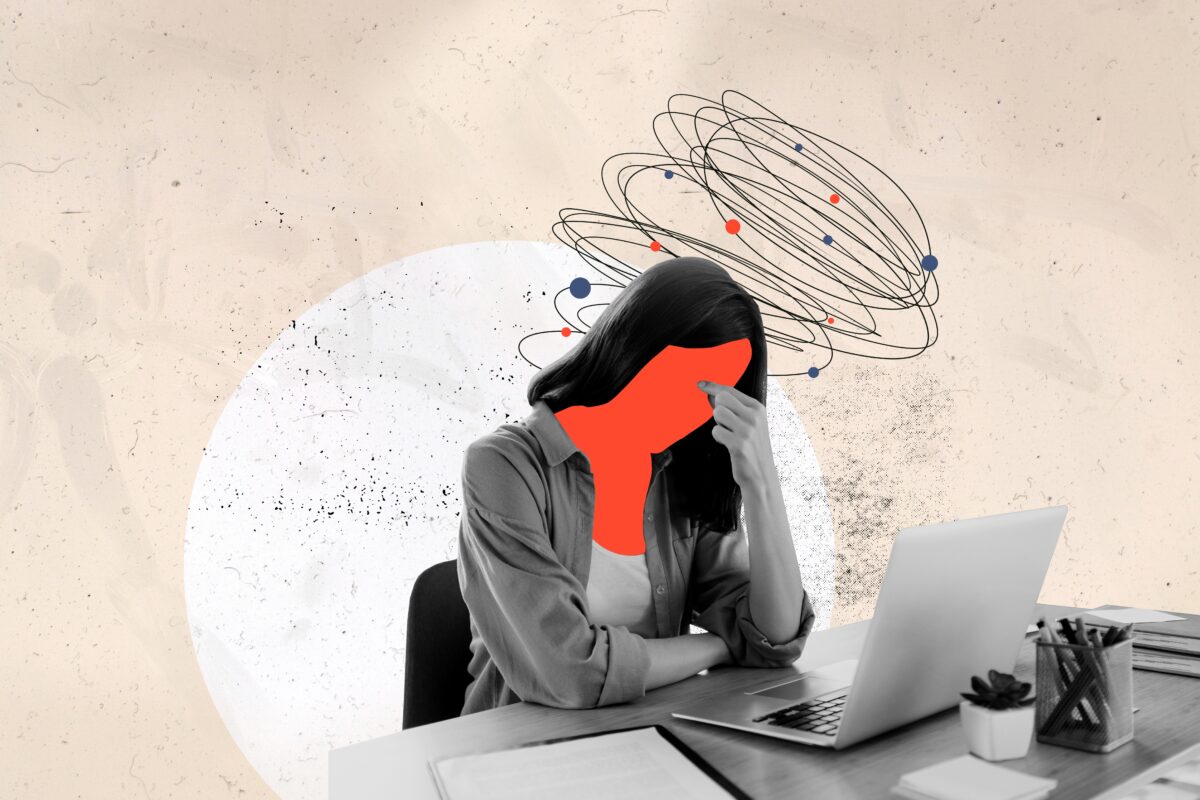
AI could increase accuracy in clinical trials
Study reveals that GPT-4 models outperform experts at selecting patients with heart failure, optimizing inclusion in clinical trials, and reducing operational costs
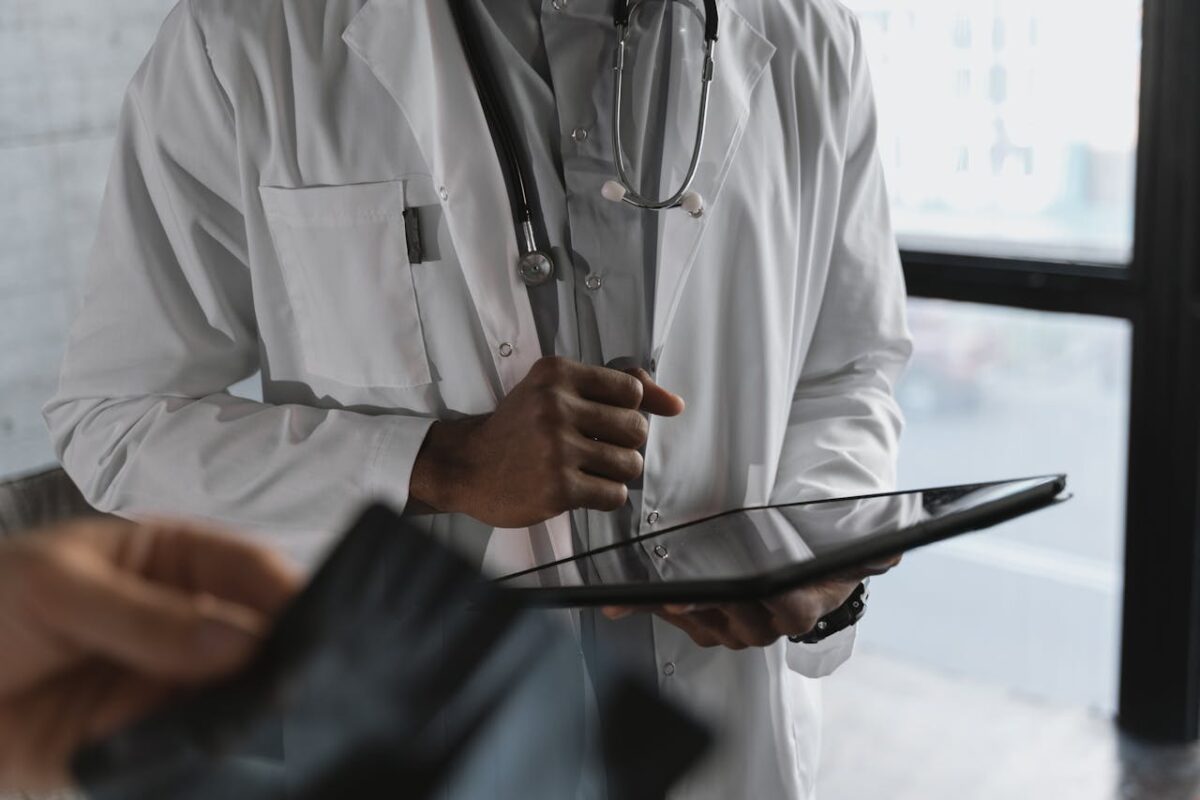
Systems based on large language models (LLMs)—a machine learning method that uses massive datasets—can significantly improve the screening of volunteers for clinical trials, automating the process and reducing costs.
The findings are described in an article published in The New England Journal of Medicine (NEJM) by researchers from Brigham and Women’s Hospital in Boston, USA.
Based on the premise that conducting a clinical trial is a laborious, error-prone process that demands significant time and resources, the authors used Generative Pre-Trained Transformer 4 (GPT-4), the fourth generation LLM by OpenAI, to carry out a pilot study as part of the Cooperative Program for ImpLementation of Optimal Therapy in Heart Failure (COPILOT-HF).
The randomized, open initiative is being implemented within the Mass General Brigham healthcare system to test remote care strategies to optimize the prescription of guideline-directed medications for patients with heart failure.
To determine patient eligibility for the study, the team developed a clinical note-based question answering system powered by retrieval-augmented generation (RAG) and GPT-4 called the RAG-Enabled Clinical Trial Infrastructure for Inclusion Exclusion Review (RECTIFIER).
The framework was used with clinical notes from three data sets of 100, 282, and 1,894 patients—with an average of 12 notes per patient—to respectively develop, validate, and test the performance of the proposed model.
In parallel with the screening performed by RECTIFIER and COPILOT-HP, a specialist physician conducted a blind review to establish “gold standard” responses to the thirteen target-patient inclusion and exclusion questions.
High precision
The results show that compared to the gold standard answers, the AI process was 97.9% accurate, while the team behind the study (which evaluated the same records) achieved 91.7% accuracy in the recruitment of patients with symptomatic heart failure for clinical trial.
The AI model also showed a sensitivity of 92.3% and a specificity of 93.9%. “This result was a surprise—better than we expected,” cardiologist Alexander Jordan Blood of Brigham and Women’s Hospital, a researcher at Harvard University School of Medicine and coauthor of the study, told Science Arena.
“We are contributing to the understanding that these new AI tools can improve the speed and accuracy of enrolling in clinical trials while lowering costs,” says Blood.
The RECTIFIER and COPILOT-HP study showed that with a single question strategy, it was possible to determine selection criteria eligibility at an average cost of 11 US cents (US$0.11) per patient, while the combined questions approach had an average cost of just 2 cents (US$0.02) per patient.
For comparison, the approximate cost of the traditional screening model is US$34.75. “This is a sign that even with less financial investment, AI can be used to make a larger number of clinical trials viable,” says Blood.
According to the researcher, the use of AI in clinical trials could speed up the process of determining whether a therapy is effective. He envisions that the trials will become cheaper and more equitable, without sacrificing safety.
Medicines developed by completing the phases of a clinical trial will thus be able to reach patients more quickly.
Potential hazards
In clinical trials, candidates are only enrolled if they meet specific (inclusion and non-exclusion) criteria associated with age, diagnoses, key health indicators, comorbidities, and current or past medications, among other factors.
These criteria help researchers to increase the likelihood that participants who can actually benefit from the treatment are included in the study.
The process also helps prevent the inclusion of patients who have unrelated health problems or who are taking medications that could interfere with the results.
The objective (of the use of GPT-4 in clinical trial screening) is to increase accuracy, efficiency, and reliability. However, there is also potential for misuse of the technology, resulting in selection prejudice and ethical and technical concerns.
Before complete automation of the screening process, the researchers stress that it is important to carefully consider all the potential hazards and implement appropriate strategies to mitigate them.
Furthermore, although the study offers insights and promising applications of GPT-4 in clinical trial screening, the findings should be interpreted in light of their context-specific nature.
As advances are made, the scientists emphasize, it is important that these technologies continue to be improved, ensuring they remain applicable across a wide spectrum of clinical scenarios and securing the continued integrity of trials.
The authors point out that the application of RECTIFIER in the medical field is not limited to clinical trials. The framework could also be used to help address gaps in the quality of care for diseases such as heart failure, optimize guideline-directed medication prescription, and assist in public health management.
*
This article may be republished online under the CC-BY-NC-ND Creative Commons license.
The text must not be edited and the author(s) and source (Science Arena) must be credited.